Blogs, Case studies and Expert perspectives
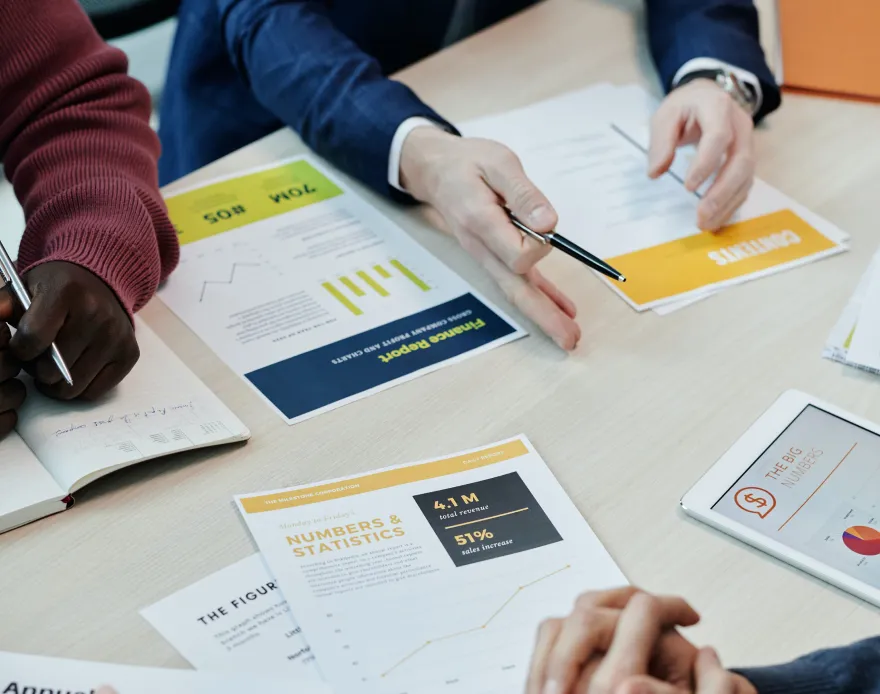
Blogs
.png)
Introduction
In the rapidly evolving landscape of healthcare, accurate medical coding is crucial. It ensures proper billing, regulatory compliance, and quality patient care. However, achieving coding accuracy can be complex and time-consuming, often prone to errors and inconsistencies.
Arintra addresses these challenges by using artificial intelligence to automatically code medical records. This revolutionary approach helps healthcare providers save time, reduce errors, and improve patient care. This blog explores how Arintra is transforming medical coding, making it more accurate and efficient.
Challenges in Traditional Coding Practices
Traditional manual coding practices in healthcare face numerous challenges. Human coders often struggle to accurately translate complex medical procedures and diagnoses into standardized codes. This process is not only time-consuming but also prone to errors and inconsistencies.
Manual coding also lacks scalability, making it difficult for healthcare organizations to keep up with the increasing volume and complexity of medical records. Additionally, manual coding relies heavily on individual coders' knowledge and experience, leading to variations in coding quality and accuracy.
The Impact of Coding Errors on Healthcare
Coding errors can have significant consequences for healthcare organizations, patients, and the overall healthcare system. Inaccurate coding can result in claim denials, delayed payments, revenue loss, and increased administrative costs.
Moreover, inaccurate coding can lead to incorrect billing, potentially resulting in overbilling or underbilling. This not only affects the financial health of healthcare organizations but also raises concerns about fraud and abuse.
From a patient care perspective, coding errors can result in incorrect treatment plans, incorrect medication dosages, and delayed or denied services. This can negatively impact patient outcomes and satisfaction.
The Need for Automation in Medical Coding
The increasing complexity of healthcare data and the need for accuracy and efficiency have led to a growing demand for automation in medical coding. AI and machine learning technologies offer the potential to streamline coding processes, reduce errors, and improve overall coding accuracy.
Automation can also help healthcare organizations address challenges such as increasing coding volumes, compliance with evolving regulations, and the need for real-time coding updates. By automating repetitive and time-consuming coding tasks, healthcare organizations can free up valuable resources to focus on more strategic initiatives.
Arintra's Approach to Autonomous Coding
Arintra's autonomous coding solution leverages AI and machine learning to automate the coding process. By analyzing medical records and clinical documentation, Arintra's technology can accurately assign appropriate codes to diagnoses, procedures, and services.
Arintra's technology is designed to adapt and learn from new data, ensuring that coding practices remain up-to-date and compliant with regulatory requirements. The platform also provides real-time feedback to coders, helping them make informed decisions and improve coding accuracy.
Benefits of Arintra's Autonomous Coding
Arintra's autonomous coding solution offers numerous benefits. By automating coding processes, Arintra helps healthcare organizations reduce coding errors, improve coding accuracy, and streamline coding workflows.
The platform also improves efficiency by reducing the time and resources required for manual coding. This allows healthcare organizations to process coding tasks faster and more cost-effectively.
Additionally, Arintra's autonomous coding solution enhances compliance by ensuring that coding practices adhere to regulatory standards and guidelines. This reduces the risk of audit failures and penalties, ensuring that healthcare organizations operate ethically and responsibly.
Future Trends in Medical Coding
Looking ahead, the future of medical coding is likely to be shaped by continued advancements in AI and machine learning technologies. These technologies will further automate coding processes, improve accuracy, and enhance efficiency in healthcare organizations.
The use of natural language processing (NLP) and predictive analytics will enable more sophisticated coding algorithms, capable of understanding and interpreting complex medical documentation. This will not only improve coding accuracy but also enable healthcare organizations to extract more meaningful insights from their data.
Conclusion
In conclusion, Arintra's autonomous coding solution represents a significant advancement in the field of medical coding. It offers a transformative approach to improving accuracy and efficiency, addressing the challenges associated with traditional manual coding practices.
By leveraging AI and machine learning technologies, Arintra helps healthcare organizations reduce errors, streamline workflows, and ensure compliance with regulatory standards. This not only improves the financial health of healthcare organizations but also enhances patient care and outcomes.
Looking ahead, the future of medical coding is bright, with automation playing a central role in redefining coding practices and improving outcomes for healthcare organizations and patients alike.
.png)
Introduction
Accurate medical coding is fundamental for the smooth operation of healthcare systems in the United States, ensuring correct billing, adherence to regulatory standards, and optimal patient care. While much attention is given to the coding process itself, the significance of audit trails is often overlooked. These trails offer a detailed chronicle of coding activities, providing transparency and accountability in the coding process. This blog delves into the crucial role of audit trails in US medical coding, highlighting their importance in ensuring accuracy, compliance, and efficiency.
Importance of Audit Trails in Medical Coding
Ensuring Accuracy and Transparency: Audit trails serve as detailed records of all coding activities, offering transparency into who accessed patient records and the changes made. This transparency is crucial for maintaining accuracy, allowing coders to track their actions and identify errors. By reviewing audit trails, coders can ensure the correctness of codes assigned to each patient's diagnosis and treatment, reducing the risk of claim denials and ensuring appropriate patient care.
Meeting Regulatory Requirements: In the US, healthcare providers must comply with various regulations to protect patient privacy and data security. Audit trails play a crucial role in meeting these regulatory requirements by providing detailed records of coding activities. They help demonstrate compliance with regulations such as HIPAA, reducing the risk of fines and penalties.
Enhancing Efficiency and Accountability: Audit trails improves the accuracy and transparency of coding processes as well as enhances efficiency and accountability. By maintaining detailed records, healthcare providers can track the progress of each coding task, identify bottlenecks, and improve processes. Additionally, audit trails promote accountability by holding individuals responsible for their actions, ensuring ethical coding practices and compliance with regulatory requirements.
The Role of Arintra's Audit Trails
Arintra, an AI-powered autonomous coding solution, offers a unique approach to audit trails in medical coding. Arintra's audit trail provides detailed insights into how codes are generated and which parts of the medical chart contribute to each code, including ICD (International Classification of Diseases) or CPT (Current Procedural Terminology) codes, and decisions regarding Evaluation and Management (E/M) levels.
.png)
Code Generation Process: Arintra's audit trail details the process of how codes are generated for each patient encounter, including the rules, algorithms, and machine learning models used. This level of detail helps in understanding the rationale behind each assigned code, ensuring accuracy and compliance with coding guidelines.
Chart Contribution Analysis: Arintra's audit trail specifies which parts of the medical chart contribute to the assignment of specific codes, identifying specific diagnoses, procedures, or symptoms documented in the chart that lead to the selection of particular codes.
E/M Level Decision Documentation: Arintra's audit trail documents the decision-making process for selecting the appropriate E/M level, including the factors considered, such as history, examination, and medical decision-making.
Overall, Arintra's audit trail provides a comprehensive and transparent view of the coding process, ensuring accuracy, compliance, and accountability. By detailing how codes are generated and which parts of the chart contribute to each code and E/M level decision, Arintra enhances the integrity of the coding process and helps healthcare providers deliver high-quality care to patients.
Conclusion
In conclusion, audit trails are crucial for ensuring accuracy, transparency, and compliance in medical coding. They provide detailed records of coding activities, helping healthcare providers track their actions, identify errors, and demonstrate compliance with regulatory standards. Arintra's AI-powered solution enhances audit trails by generating detailed and explainable records, facilitating documentation, and integrating seamlessly with EHR systems. By leveraging Arintra, healthcare providers can improve coding practices, reduce errors, and enhance patient care.

Introduction
Prior to 1980, coding was a paper-based, time-consuming, error-prone, and inefficient process with limited practical alternatives. This soon changed with the 1980s technology boom, which brought about a protracted shift to ICD-10-CM/PCS and fundamentally changed the process and scale of gathering and managing patient data.
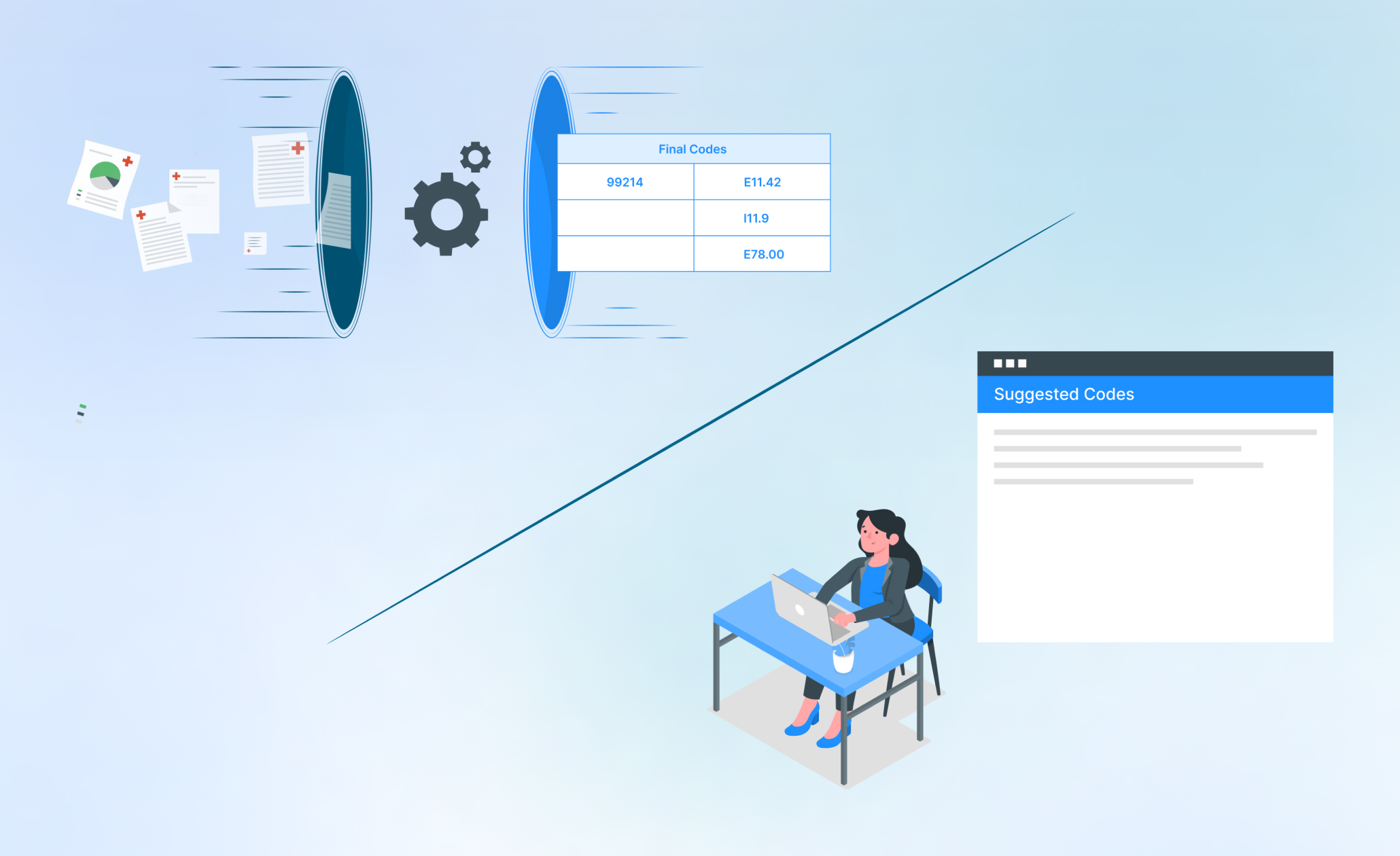
It is essential to draw a distinction between simple, primitive solutions like computer-assisted coding (CAC) and true automation. CAC tools primarily affect productivity and workflow, but fail to completely automate the processes. On the contrary, autonomous coding emulates human intelligence by combining large datasets with computational power and sophisticated algorithms, enabling the coders to instead address more complex RCM concerns.
CAC has proven successful in highly structured and smaller records, such as radiological tests, laboratory exams, and emergency room visits, but delivers inefficient results with larger records. On the other hand, autonomous coding has shown demonstrable results in radiology, primary care, emergency, urgent care, pathology, and other high-volume outpatient encounters. Vaccines, cardiac rehab, gastrointestinal, women's health, and outpatient therapies, are other areas with the potential to be future case types for autonomous coding.
The implementation of the CAC software can often be challenging. Subsequently, the coding specialists are also required to receive training on operating the software and must modify their existing coding procedures to conform to the new CAC workflow. In contrast, the autonomous coding software can be seamlessly implemented into the existing workflow without any modification or human intervention.
Clinical notes are often unstructured, with complex medical terminology and spelling errors. Another notable disadvantage of CAC is the inability to decipher and translate such free text into precise medical codes, resulting in error-prone, inaccurate, and unreliable coding suggestions. Autonomous coding’s robust, cutting-edge technology is the complete solution for an impressive return on investment, a streamlined revenue cycle, error-free coding, improved accuracy, and increased efficiency in the complex medical coding industry.
Case Studies
.png)
Client Overview
The client, a leading healthcare provider in the United States, serves a diverse patient population with a commitment to quality care and regulatory compliance. Facing the complexities of regulatory standards, particularly HIPAA, the organization sought solutions to streamline its coding processes and enhance compliance efforts.
Challenges Faced
1. HIPAA Compliance: The organization grappled with the intricate requirements of HIPAA, especially concerning the protection of electronic protected health information (ePHI). Manual coding processes made it challenging to maintain compliance and track access to sensitive patient data.
2. Audit Trail Documentation: The lack of a robust system for documenting audit trails related to coding activities posed a significant challenge. This deficiency hindered the organization's ability to demonstrate compliance and respond effectively to audits.
3. Coding Accuracy: Ensuring coding accuracy was paramount not only for billing accuracy but also for regulatory compliance. Manual processes were prone to errors, increasing the risk of non-compliance and financial penalties.
Arintra's Solution
Arintra's AI-powered autonomous coding solution offered a comprehensive solution to the organization's compliance challenges. By leveraging advanced algorithms and machine learning, Arintra provided detailed audit trails and ensured coding accuracy, helping the organization meet HIPAA requirements and improve operational efficiency.
Implementation
The implementation of Arintra's solution involved close collaboration between the organization's compliance, IT, and coding teams. Arintra was configured to generate detailed audit trails for all coding activities, providing transparency into the coding process and ensuring compliance with HIPAA guidelines. Extensive training sessions were conducted to familiarize staff with the new system and ensure seamless integration into existing workflows.
Results
1.Improved Compliance: Arintra's detailed audit trails enabled the organization to demonstrate compliance with HIPAA regulations. The system tracked all coding activities, ensuring that ePHI was protected according to HIPAA standards.
2. Enhanced Audit Preparedness: Arintra's documentation of audit trails improved the organization's ability to respond to audits efficiently. Auditors could easily review the audit trails to verify compliance, reducing the risk of penalties.
3. Increased Coding Accuracy: Arintra's advanced algorithms significantly improved coding accuracy, reducing errors and ensuring compliance with coding guidelines. This improvement not only supported accurate billing but also enhanced overall operational efficiency.
Conclusion
Arintra's AI-powered autonomous coding solution proved instrumental in helping the healthcare provider ensure compliance with regulatory requirements, particularly HIPAA. By implementing Arintra, the organization improved its compliance efforts, enhanced audit preparedness, and increased coding accuracy, ultimately leading to better patient care and operational excellence.
.png)
Client Overview
The client is a large healthcare organization consisting of a health system, a management services organization (MSO) with 3800 providers, and a prominent physician group. The organization provides a wide range of healthcare services to patients across the United States.
Challenges Faced
Inefficient Coding Processes: The organization's manual coding processes were time-consuming and prone to errors. Coders often struggled to keep up with the volume of coding required, leading to delays in claim submission and reimbursement.
Integration Issues: The organization's existing EHR system did not fully integrate with their coding processes. This lack of integration resulted in disjointed workflows, as coders had to switch between systems to access patient information and assign codes.
Compliance Concerns: Ensuring compliance with regulatory standards, such as HIPAA, was challenging due to the manual nature of the coding processes. The organization needed a solution that would automate compliance checks and ensure that coding practices met regulatory requirements.
Arintra's Solution
Arintra's AI-powered autonomous coding solution offered a comprehensive solution to the organization's challenges. By seamlessly integrating with their existing EHR system, Arintra streamlined the coding process, reducing errors and improving efficiency.
Implementation
The implementation of Arintra's solution involved collaboration between the organization's IT and coding teams. Arintra was configured to work with the organization's existing EHR system, ensuring a seamless integration process. Training sessions were conducted to familiarize staff with the new system and ensure that they could use it effectively.
Results
Improved Efficiency: Arintra's integration with the EHR system significantly reduced the time taken for coding. Coders were able to complete coding tasks more quickly, allowing them to focus on more complex cases that required their expertise.
Reduced Errors: The automation provided by Arintra led to a reduction in coding errors. The system's advanced algorithms helped coders assign codes more accurately, reducing the risk of claim denials and ensuring that patients received the appropriate care.
Streamlined Workflows: Integration with the EHR system streamlined workflows, eliminating duplication of efforts. Coders no longer had to switch between systems to access patient information, improving overall operational efficiency.
Enhanced Compliance: Arintra's detailed audit trails and compliance features ensured that the organization remained compliant with regulatory standards. The system automatically checked codes against regulatory guidelines, reducing the risk of fines and penalties.
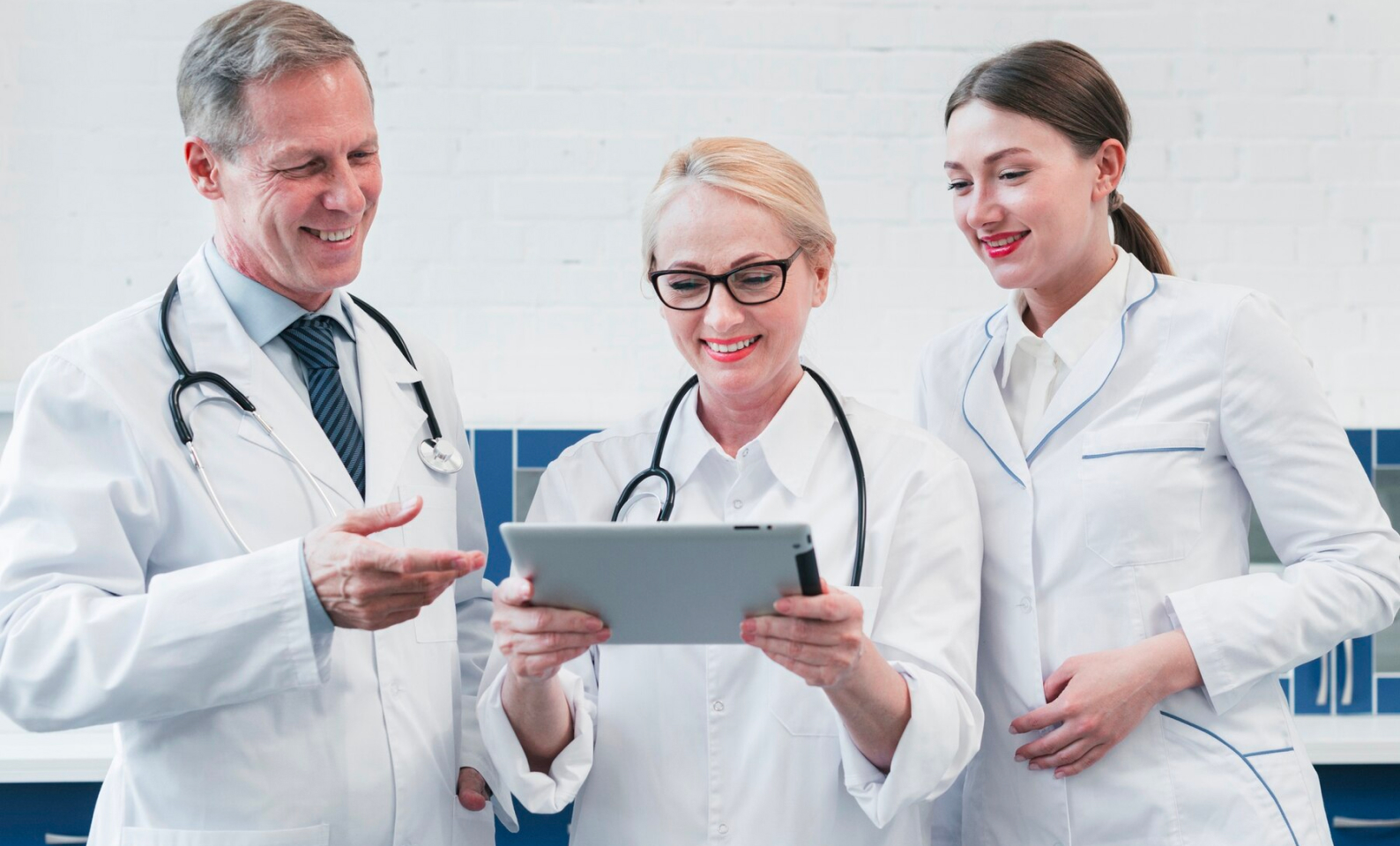
Overview
A large provider organization in the United States, with a monthly visit volume of 231,360, faced several challenges in their revenue cycle. Adopting Arintra's AI powered autonomous medical coding solution brought a significantly positive impact from November 1 to November 30, 2022, marking a transformative change for the organization.
Challenges
- 75% physician coded charts were billed directly
- 10% denial rate
- High volume low dollar claims
- Massive coding backlogs
- Shortage of medical coders
These challenges collectively resulted in an annual loss of $12 million for the provider organization.
Solution
Arintra’s powerful autonomous medical coding technology addressed these multifaceted challenges by leveraging deep learning, NLP, clinical LLMs and generative AI. The seamless and secure bi-directional integration with the provider organization’s existing EHR required zero IT lift and ensured zero workflow change. Arintra autonomously processed every patient chart, consistently applied business rules and generated precise direct-to-billing charges and codes. It automated an impressive 696 charts per hour, significantly expediting the coding process. By automating the routine coding tasks, Arintra freed providers from coding workload and gave them more time to provide optimal patient care.
Results
Arintra addressed all the challenges and increased the average monthly revenue by 4% for the large provider organization. A significant 41% drop in claim denial rate showcased Arintra's prowess in enhancing coding accuracy. Moreover, Arintra's implementation led to a commendable 22% reduction in coding backlogs, ensuring a smoother workflow.
Book a demo now
To experience the power of autonomous coding and learn how to achieve immediate ROI
Coding accuracy
Reduced coding denials
Time saved for providers
Reduced A/R days
Cost savings
Faster turnaround time
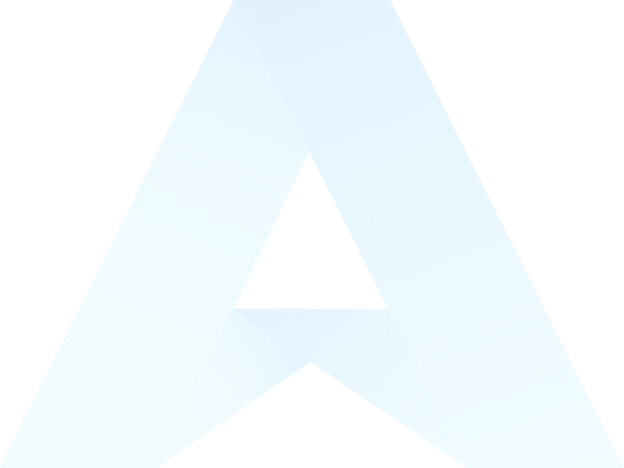